Portfolio Backtesting Mistakes That Skew Results
Backtests can mislead if they ignore execution costs, regime shifts, or survivorship bias—test smarter, not just harder.
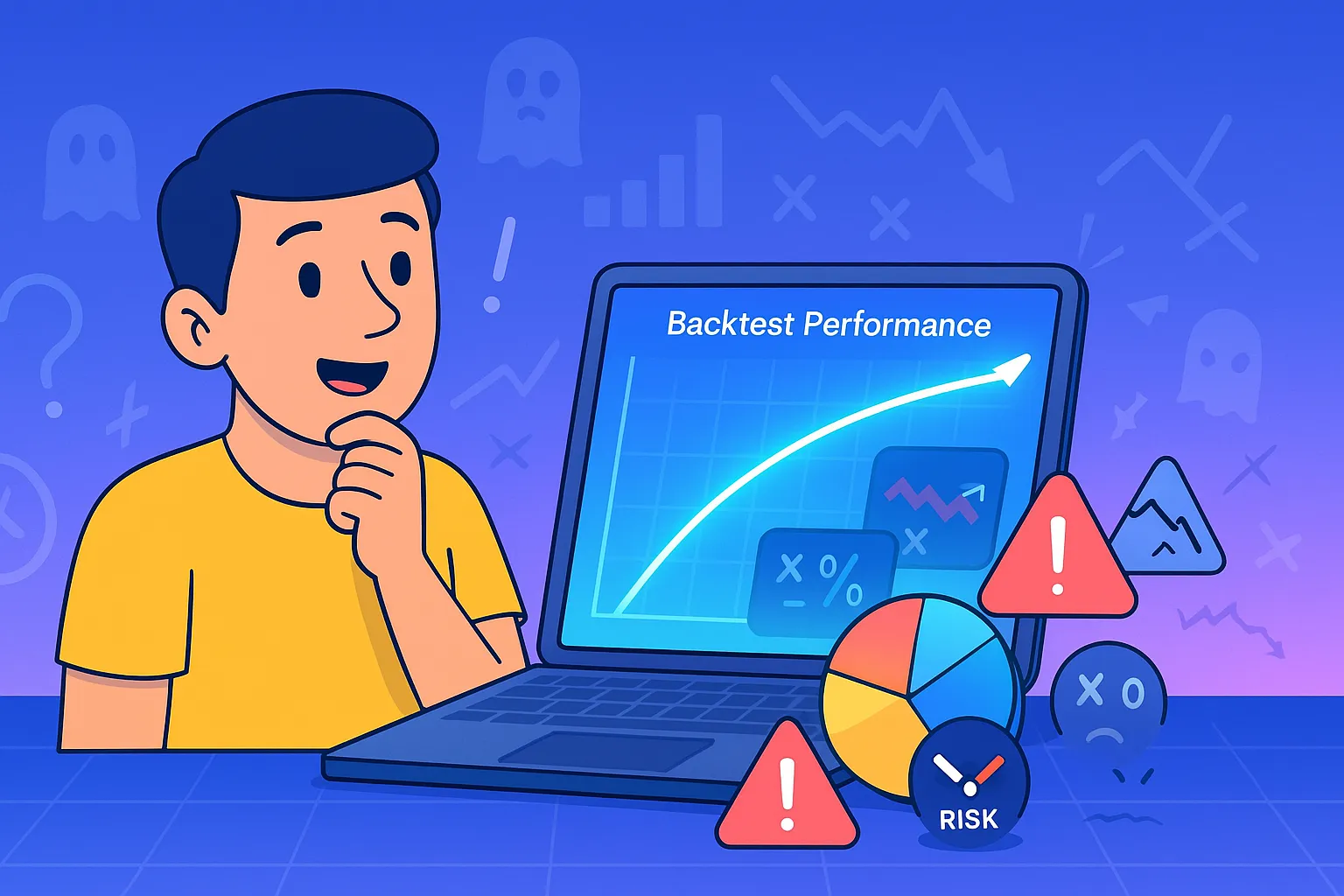
This content has been reviewed and edited by an Investment Advisor Representative working for Global Predictions, an SEC-registered Investment Advisor.
If you've ever run a backtest and thought, "Wow, this strategy is a goldmine!"—you’re not alone. Backtesting feels like an investing cheat code: just plug in historical data, fine-tune the parameters, and watch your portfolio thrive over the years. But here’s the problem: most backtests give a false sense of security because they don’t reflect how a strategy will actually perform in the real world.
The truth is, all backtesting is flawed in some way. Survivorship bias, unrealistic execution assumptions, and market shifts can all distort outcomes, leading investors to trust strategies that might not work going forward.
So, how do you backtest properly? Let’s go over the most common portfolio backtesting mistakes, why they happen, and how to make your tests as reliable as possible.
Key Takeaways
- Backtesting isn’t perfect—historical performance doesn’t guarantee future results.
- Survivorship bias, unrealistic execution costs, and overfitting can make backtests misleading.
- Market conditions change, so a strategy that worked in one period may fail in another.
- Robust backtesting methods focus on real-world execution and adaptability.
Mistake #1: Survivorship Bias
What It Is: Survivorship bias happens when backtests only include assets that exist today, ignoring the ones that failed or disappeared. This skews results because failing stocks, funds, or companies are removed from the dataset, making past performance look better than it really was.
- Hypothetical Example: Imagine you’re testing a strategy that invests in top-performing mutual funds over the last 20 years. If your dataset only includes funds that still exist today, you’re ignoring the fact that many funds were closed or merged due to poor performance. Your backtest will likely overestimate returns because it’s missing the losers.
It’s like trying to pick a winning sports team by only looking at championship winners—while ignoring the dozens of teams that never made it past the first round.
How to Fix It:
- Use a survivorship-bias-free database that includes historical records of delisted stocks and defunct funds.
- Include failed investments in the test to get a more realistic picture of past performance.
- Look beyond just the winners—study what went wrong with the losers to avoid potential pitfalls.
Mistake #2: Overfitting to Historical Data
What It Is: Overfitting happens when a backtest is too closely tailored to past data, leading to strategies that work beautifully on historical charts but fail in live markets.
- Hypothetical Example: Let’s say you develop a trading strategy based on specific conditions from the 2010-2020 bull market—maybe it buys tech stocks after three consecutive down days. It looks great in backtests, but in a different market environment (e.g., a bear market or rising interest rate cycle), it might fail completely.
How to Fix It:
- Test across multiple time periods with different market conditions.
- Use out-of-sample testing—train your model on one dataset and test it on another.
- Focus on broad principles rather than hyper-specific rules.
Mistake #3: Ignoring Market Regime Shifts
What It Is: Market conditions aren’t static—a strategy that worked well in one economic environment may break down in another. Many backtests fail because they assume markets behave consistently over time.
- Hypothetical Example: A momentum strategy that performed well from 2009-2021 (a long bull market with low interest rates) might struggle in a high-inflation or recessionary environment, like the one seen in 2022. Market structure changes, and your backtest needs to account for that.
How to Fix It:
- Test your strategy in multiple market regimes (bull, bear, high inflation, low volatility, etc.).
- Use rolling backtests that test performance over different economic cycles.
Mistake #4: Unrealistic Execution Assumptions
What It Is: Backtests often assume perfect trade execution—that you’ll always be able to buy and sell at the closing price with no slippage, no spreads, and no liquidity issues. In reality, this is rarely the case.
- Hypothetical Example: A backtest might assume you can buy shares of a small-cap stock at the exact price shown on historical data. But in practice, thinly traded stocks have large bid-ask spreads and limited liquidity, making execution much more difficult (and costly) than the backtest suggests.
How to Fix It:
- Include trading costs, slippage, and liquidity constraints in your backtest.
- Simulate realistic order execution based on average daily volume.
Mistake #5: Cherry-Picking Time Periods
What It Is: If you only backtest over timeframes where your strategy worked well, you’re ignoring the periods where it failed.
- Hypothetical Example: A strategy might look fantastic if tested from 2010-2020, a period of mostly rising markets. But what happens if you test it from 2000-2010, a period with two major crashes? If a strategy only performs well in certain handpicked timeframes, it may not be robust.
How to Fix It:
- Test over multiple time periods, including bear markets and economic downturns.
- Avoid curve-fitting—your strategy should work across different conditions, not just cherry-picked ones.
How to Backtest a Portfolio the Right Way
Backtesting should be more than just looking at past returns—it should reflect how a strategy would perform in different market conditions. A solid backtest doesn’t just assume a static market; it tests variations in volatility, liquidity, and macroeconomic factors to see how a strategy holds up in the real world.
For example, instead of assuming a steady bull market, ask:
- How does the strategy perform during sudden interest rate hikes?
- What happens if a major asset class experiences a sharp drawdown?
- How does liquidity change in stressful market environments?
By incorporating these tests, you ensure that your backtest isn’t just a rosy picture of the past, but a stress-tested model for the future.
Now that we’ve covered the common mistakes, here’s how to run a more reliable backtest:
- Use a broad dataset that includes failed stocks, delisted assets, and different market cycles.
- Account for trading costs and liquidity issues—don’t assume perfect execution.
- Run multiple scenario tests—don’t just test in bull markets.
- Avoid overfitting by keeping strategies simple and adaptable.
- Stress-test your strategy in different economic environments.
By following these steps, you’ll develop backtests that better reflect real-world results and help you make smarter investment decisions - but even then, remember that all backtests are flawed!
FAQ
1. What is portfolio backtesting?
Portfolio backtesting is the process of testing an investment strategy using historical data to see how it would have performed in the past. It helps investors evaluate potential strengths and weaknesses before committing real money.
2. Why can backtesting be misleading?
Backtesting can give false confidence if it doesn’t account for factors like survivorship bias, overfitting, market regime shifts, and execution costs. A strategy that worked in the past may not perform well in future conditions.
3. How can I make my backtests more realistic?
To improve accuracy:
- Use a broad dataset that includes failed stocks and economic downturns.
- Account for trading costs, liquidity, and slippage.
- Test across different market conditions, not just bull markets.
4. Should I rely solely on backtesting to make investment decisions?
No. While backtesting is a valuable tool, it should be used alongside risk management, forward-looking analysis, and stress testing to create a well-rounded investment strategy.
5. How often should I update my backtesting models?
Regularly review your backtests, especially when market conditions change significantly. A strategy that worked five years ago might not hold up in today’s economic environment.
How optimized is your portfolio?
PortfolioPilot is used by over 30,000 individuals in the US & Canada to analyze their portfolios of over $30 billion1. Discover your portfolio score now:
Analyze your entire net worth
360° portfolio analysis, AI Assistant, and personalized recommendations guided by our Economic Insights Engine.

Which Portfolio Analysis Tool Is Right for You?
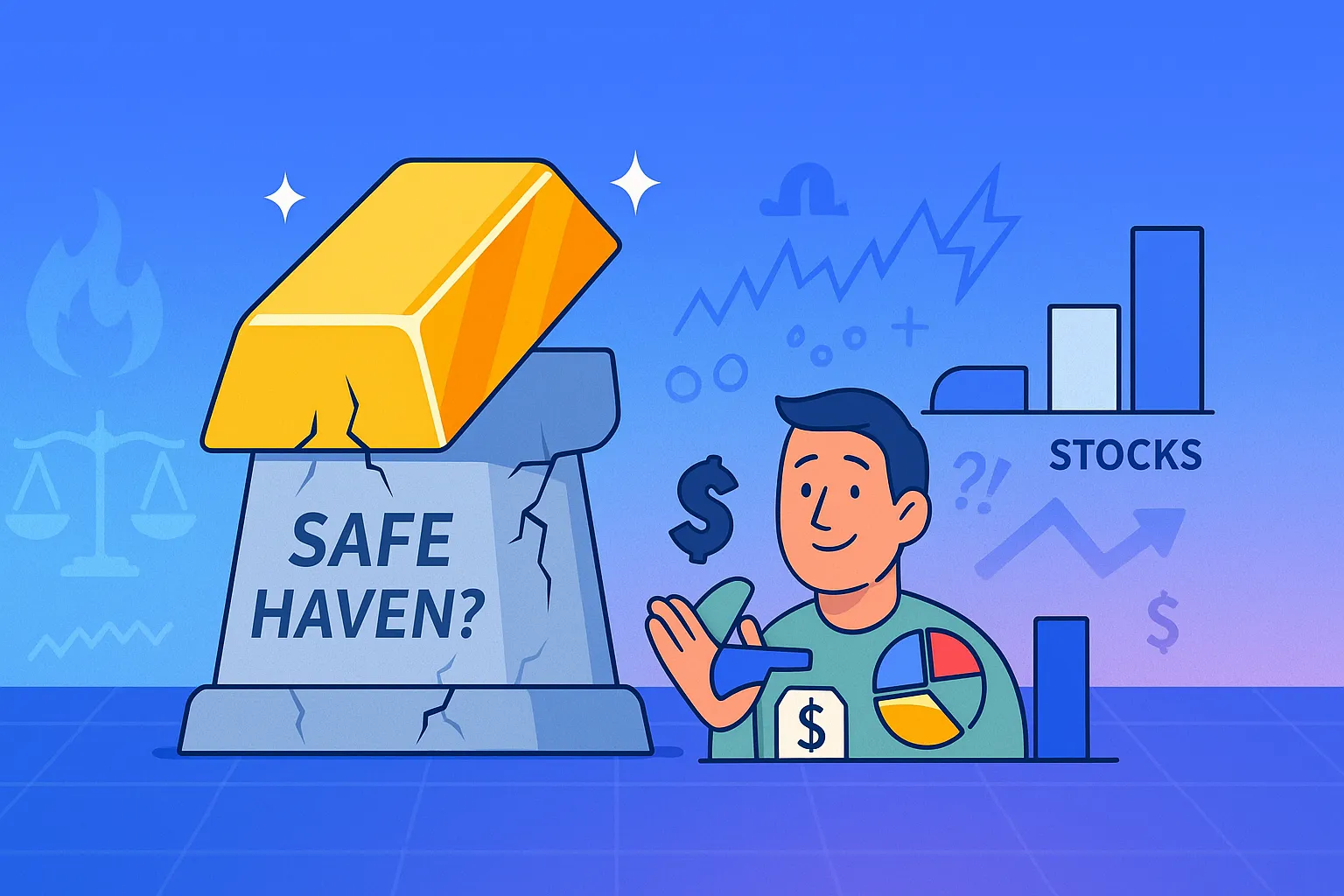
Gold Can be a Terrible Investment – Here’s Why
